As AI-generated imagery becomes more prevalent, concerns about bias and fairness in the representation of human subjects have been a recurring concern among responsible tech practitioners and society at large. Various studies [1,2,3] show that many large-scale text-to-image models (T2Is) reinforce societal stereotypes, under or mis-represent certain demographic groups, and fail to align with input prompts in socially sensitive domains. To address these challenges, a team of researchers from TikTok AI Governance team has introduced a new framework designed to assess fairness in AI-generated images from different text-to-image models.
Fairness in the field of machine learning refers to the pursuit of reducing algorithmic bias in decision making processes. When it comes to image generation, such as for pictures of human faces, these decisions are highlighted by the choice of several visual aspects such as accessories, facial expressions, context, lighting, skin texture, and skin color, among others. The images are based on a textual prompt that sometimes contain demographic information like "a female lawyer," "Asian firefighter," and more. When prompts do not carry descriptive information, like gender, occupation, race or other demographics, the models interpret the text and have to make a decision on which demographics to represent, which in turn allows the implicit bias of image generation of large-scale text-to-image models to be accurately measured.
There are many frameworks that analyse the fairness of the resulting image produced by T2I, but TikTok's recent model—coined INFELM (short for In-depth Fairness Evaluation of Large Text-To-Image Models)—scored a higher skintone precision and a stronger bias-sensitive alignment, considering demographic context and ethical societal values in comparison to previous works on the same topic.
Method
To develop this framework, researchers created 246 prompts in partnership with experts participating in the Product Equity Working Group of the Tech Accountability Coalition, an initiative managed by The Aspen Institute. The prompts were developed based on six different bias-sensitive domains to generate images as the basis to measure generation alignment, and depict the content demographic distribution of these prompts. Each prompt generated 100 images to be compared and aggregated as demographic models. Once everything was created, the results were then compared and measured for representation biases and alignment errors with actual demographics from authoritative sources such as the US Census Bureau.
While fairness and biases may be subjective and vary in different cultural contexts and ethical perspectives, this research project takes a mathematical stance to determine bias based on a 20% variance (or mismatch) between expected distribution (for example, those of the US. Census Bureau) and actual model distribution (aggregated model).
Contributions
INFELM introduces an advancement in bias detection and fairness assessment for text-to-image models. Unlike existing audits, which often rely on subjective evaluations or broad statistical trends, INFELM introduces:
- A Precision-Enhanced Skintone Classification Model: Utilizing facial topology and refined skin pixel representation, INFELM improves classification accuracy by 16.04%, ensuring more precise demographic analysis.
- Bias-Sensitive Content Alignment Metrics: Evaluates whether AI-generated images faithfully represent the intent of text prompts, particularly in contexts related to gender, race, and professional identity.
- A Generalizable Representation Bias Framework: Offers systematic measurements of demographic representation, highlighting disparities across different population groups.
- Comprehensive Model Testing Across Socially Sensitive Domains: INFELM has been applied to multiple large-scale text-to-image models, uncovering persistent patterns of bias that impact real-world AI applications.
An Improved Standard for AI Fairness Evaluation
This research-driven approach provides a structured methodology for evaluating representation bias, content alignment, and demographic inclusivity in multimodal AI systems. The framework can positively impact the field of AI systems in several ways. Firstly, it enables teams of AI Developers to evaluate and mitigate bias before deploying text-to-image models in consumer applications. Secondly, it allows regulators and policymakers to establish a quantifiable benchmark for fairness in AI-generated content, supporting ethical AI governance. Lastly, it helps industry leaders and communities to ensure compliance with fairness and accountability standards, building trust and safety in AI-driven creative tools.
As AI-generated content continues to shape digital experiences, frameworks like INFELM may be critical for ensuring inclusivity, reducing harm, and fostering transparency. By introducing a rigorous evaluation methodology, TikTok Innovation is setting a new precedent for fairness in multimodal AI.
For more information on INFELM and its applications, please read the pre-print or contact the open source team.
References
[1] Bianchi, Federico, et al. "Easily accessible text-to-image generation amplifies demographic stereotypes at large scale." Proceedings of the 2023 ACM Conference on Fairness, Accountability, and Transparency. 2023.
[2] Isabel O Gallegos, Ryan A Rossi, Joe Barrow, Md Mehrab Tanjim, Sungchul Kim, Franck Dernoncourt, Tong Yu, Ruiyi Zhang, and Nesreen K Ahmed. Bias and fairness in large language models: A survey. Computational Linguistics, pages 1–79, 2024.
[3] Ninareh Mehrabi, Fred Morstatter, Nripsuta Saxena, Kristina Lerman, and Aram Galstyan. A survey on bias and fairness in machine learning. ACM computing surveys (CSUR), 54(6):1–35, 2021.
Read the full paper.
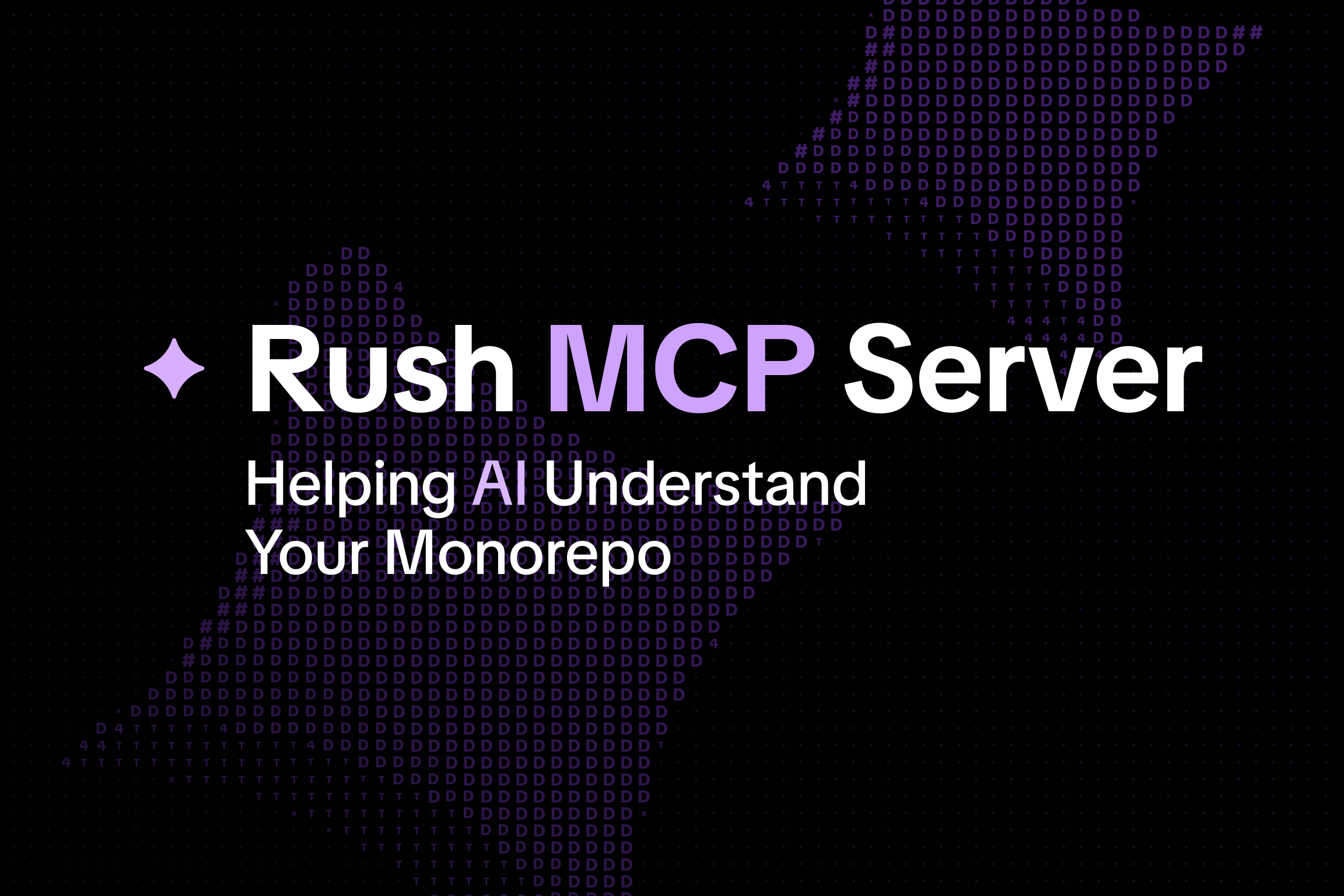
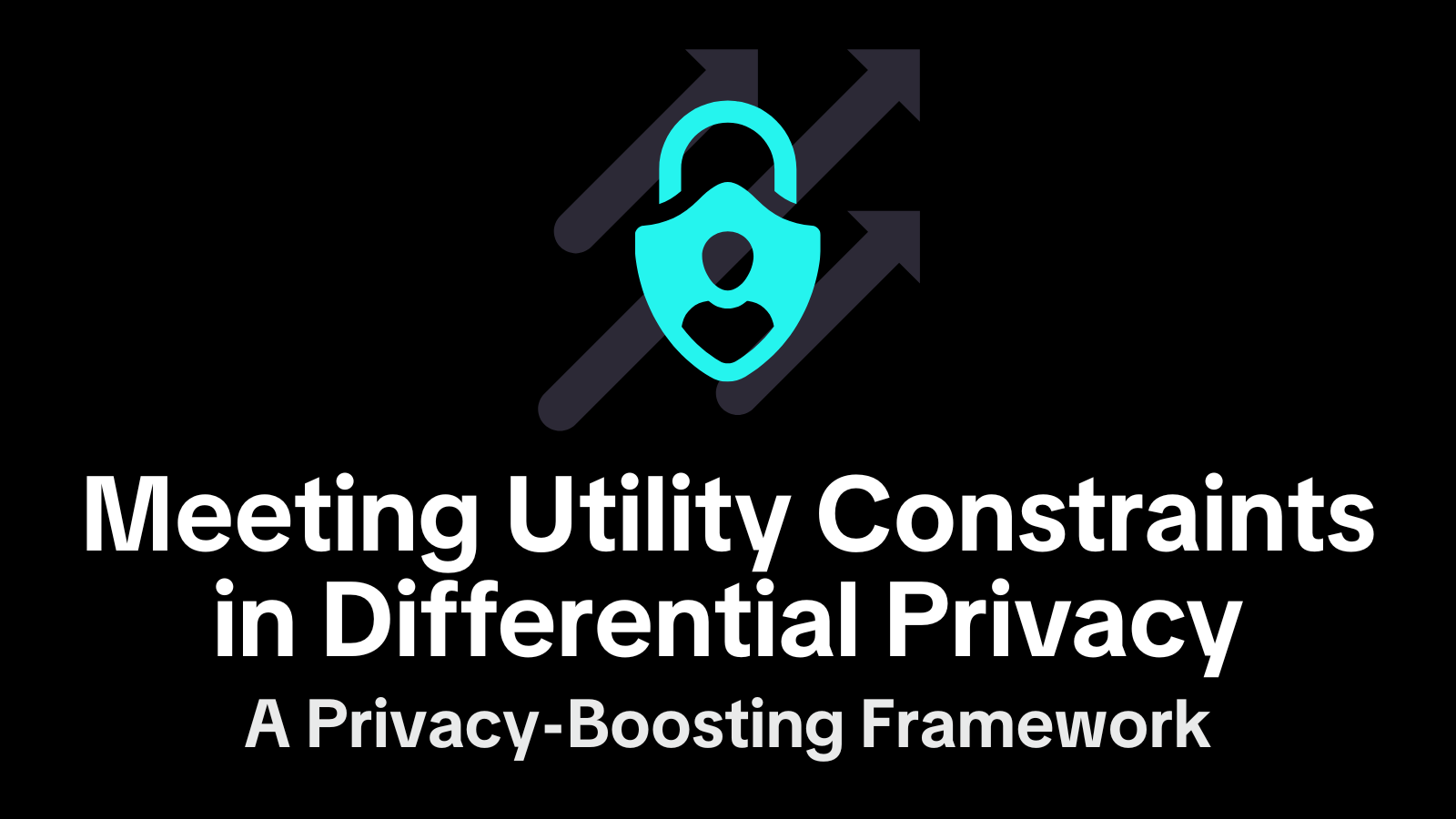
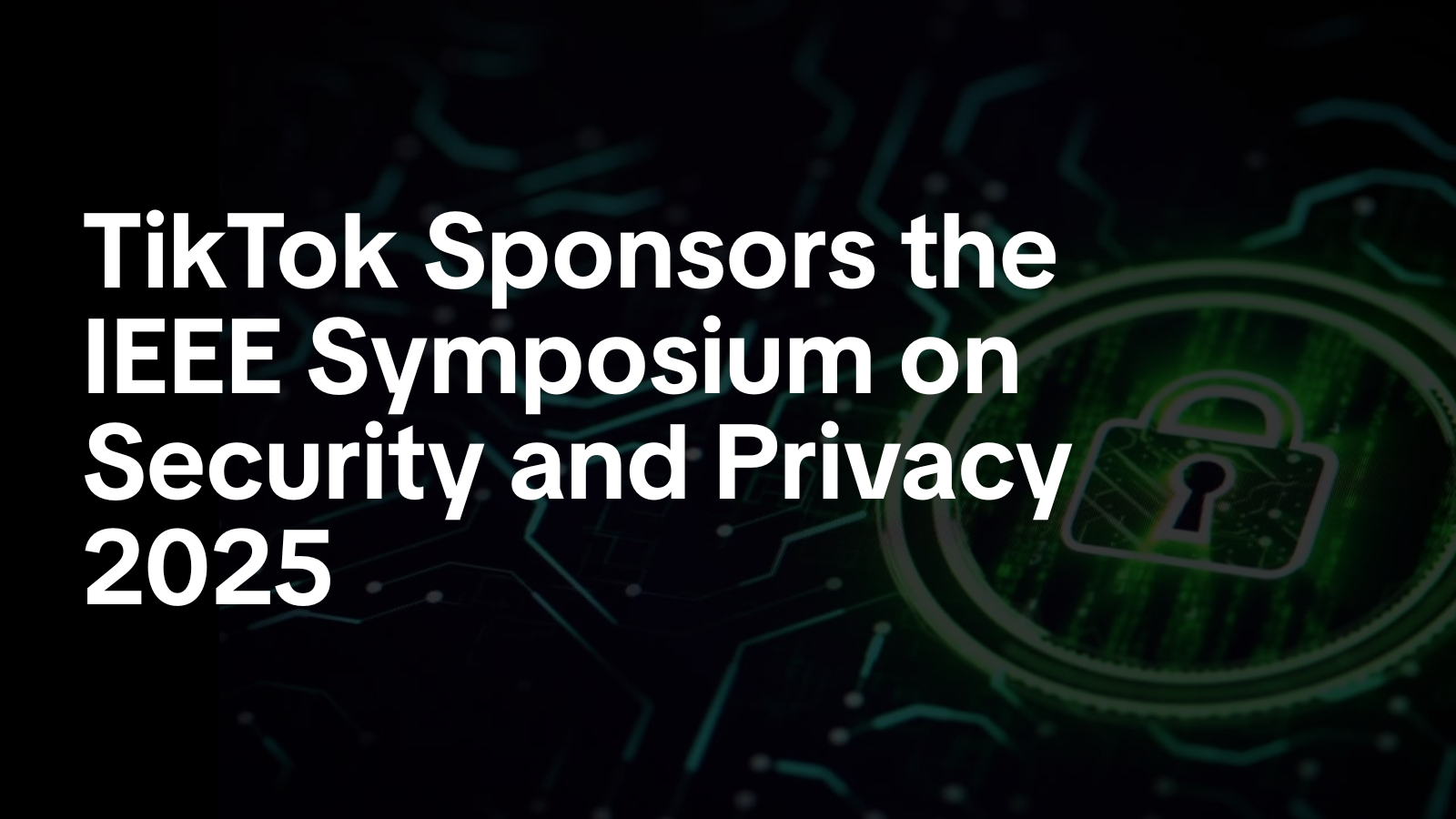
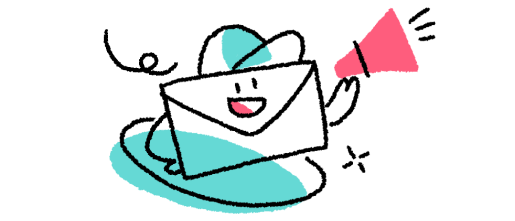