I. Motivation of Synergistic PETs
Recognizing the limitations of relying solely on a single Privacy-Enhancing Technology (PET) in privacy-sensitive scenarios, TikTok led the initiative PrivacyGo that leverages synergistic PETs fusion to enhance privacy protection.
Privacy-enhancing technologies (PETs) play a crucial role in facilitating increased data sharing while minimizing the risks to privacy and security through innovative technological advancements. One example is secure multiparty computation (MPC), which enables entities to share insights about their data without revealing the actual data itself. Companies and researchers have embraced and relied upon MPC protocols to securely share sensitive information.
To illustrate how MPC works, consider a research institute that gathers data on the lifestyle of cancer survivors, and a health insurance company that possesses claims data of its customers, including hospitalization days and healthcare usage, among other details. Both entities want to share their private data to develop an algorithm to evaluate the correlation between lifestyle choices and cancer relapse rates. They can leverage MPC to collaborate on analyzing and deriving valuable insights from their separately encrypted datasets without exposing the individual-level data.
However, recent studies have uncovered potential privacy leakage within MPC protocols. While these protocols were designed to ensure that only aggregated statistics are revealed, they are vulnerable to rogue participants seeking to exploit the system. If one of the parties involved in the MPC protocol acts curiously, they can uncover more information than previously anticipated. This newfound privacy leakage results in the curious party knowing a portion of who in their dataset is also in the other party’s dataset. To illustrate this point, consider the health data scenario mentioned earlier. This means that the health insurance company could know a portion of users in their system who are also cancer survivors. Such knowledge could be potentially utilized to make unfavorable decisions such as denying coverage or increasing premiums for those individuals.
Since the implications of such privacy leakage could be grave, it is important for practitioners to recognize the limitations of directly applying such MPC protocols in privacy-sensitive scenarios. To address these challenges and ensure robust privacy protection, TikTok has launched a new privacy initiative named PrivacyGo, which mitigates privacy leakage risks through PETs fusion.
II. PrivacyGo: TikTok’s Initiative in PETs Fusion
Each PET offers its own set of tradeoffs concerning privacy, utility, and efficiency.
PrivacyGo strives to carefully design approaches that harness the strengths of each PET while mitigating their individual limitations.
PETs (Privacy-Enhancing Technologies) encompass a wide range of technologies, including DP, MPC, TEE, k-anonymity, zero-knowledge proof, and others that span various disciplines such as information theory, cryptography, secure hardware, etc. Each PET has its own set of tradeoffs concerning privacy, utility, and efficiency, thus forming a trilemma. The integration of multiple PETs offers the potential to bring together the best of both worlds, but it is a rather complex endeavor. For instance, integrating DP and MPC would require DP to calibrate appropriate random noise within an encrypted MPC context. Simply combining PETs is insufficient to eliminate drawbacks and preserve their respective advantages. PrivacyGo, TikTok’s initiative in PETs fusion, strives to carefully design approaches that harness the strengths of each PET while mitigating their individual limitations. As a platform, TikTok is used by billions of users on a global scale. When we build products and features, securing the privacy of our users is at the forefront of our engineering strategy. Through integration of PETs, we aim to achieve a synergistic outcome that effectively balances privacy, utility, and efficiency.
III. DPCA-PSI: A Concrete Example of PETs Fusion in PrivacyGo
DPCA-PSI, a concrete example of PETs fusion in PrivacyGo, elevates privacy protection by synergistic integration of MPC and DP and finds practical application in advertising campaign measurements.
What is DPCA-PSI?
We have developed the DPCA-PSI protocol to mitigate privacy leakage in scenarios such as the health data sharing example in Section I. Private Set Intersection (PSI) is one of the important and well-studied MPC protocols, which allows a set of parties, each holding a private input set, to compute their intersection.
DPCA-PSI organically integrates a PSI protocol with a two-party differentially private (DP) mechanism. We have carefully crafted DPCA-PSI to ensure secure and efficient computation of intersection-related statistics from private datasets while maintaining DP guarantees. Our DPCA-PSI offers three significant contributions to the state-of-the-art in this field:
Figure 1. Illustration of our DPCA-PSI protocol.
- Less-revealing PSI: In practical scenarios, each party’s data records often contain multiple keys that need to be matched, leading to an exponential increase in the number of revealed intersection sizes. Our new PSI protocol reduces the number of revealed intersection sizes from exponential to linear growth, which in turn reduces the inference or linkability that can be made on the membership.
- IntersectMask DP: We complemented our less-revealing PSI with a novel distributed DP mechanism where two parties perform analysis of their intersection size while preserving privacy for both datasets. We name this two-party DP mechanism as IntersectMask Mechanism, which requires adding carefully crafted dummy variables to each party’s data records, further protecting the linear number of revealed sizes in our less-revealing PSI.
- Efficient DP: Our DP only induces small communication and computational overhead to ensure that subsequent intersection-related computations are less noisy. Our tight DP composition maximizes the number of intersect operations within a given DP privacy budget. The ratio between the data size after our protocol and that of the original data is 1.007:1, allowing for more accurate downstream computation on the intersection results.
Case Study: DPCA-PSI ensures privacy for advertising measurement
For measuring the effectiveness of advertising campaigns, ad publishers and advertisers need to share their privileged data privately. One party holds identifiers corresponding to its users who have viewed the advertising campaign, while the other party holds its identifiers and numeric values related to their users who have made purchases and corresponding spending amounts. Due to increasingly stringent privacy requirements, ads publishers and advertisers are facing challenges to measure ad conversions when they can no longer leverage user data collected from outside their platforms. The ad industry has turned to PSIs to perform ad measurement without sharing unencrypted data. However, as mentioned earlier, these protocols suffer from membership leakage, which allows advertisers or publishers to learn users’ activity on the other platform. Our DPCA-PSI method effectively mitigates such risks, ensuring user privacy and adhering to relevant privacy requirements.
IV. Stepping Forward
PrivacyGo strives to safeguard the privacy and security of TikTok users and business partners through an intricate and innovative fusion of various PETs within real-world applications.
Aside from the health data scenario and the ad measurement scenario above, our DPCA-PSI solution can be useful in a range of scenarios where privacy is paramount. For example, it can aid in detecting money laundering by comparing transactions across various financial institutions without revealing the identities of the customers involved.
Recognizing the importance of collaboration and transparency, we open source PrivacyGo(https://github.com/tiktok-privacy-innnovation/PrivacyGo), making it readily available to researchers and practitioners. PrivacyGo strives to seamlessly integrate various PETs, such as DP, MPC, homomorphic encryption, and artificial intelligence methods to enhance user privacy protection.
PrivacyGo represents one of the initiatives made by Privacy Innovation at TikTok to research innovative ways of safeguarding the privacy and security of our users and protecting our partner organizations’ sensitive information, all while leveraging the benefits of cutting-edge technologies.
PrivacyGo demonstrates the intricate and innovative fusion of various PETs, showcasing that with dedicated engineering effort, it is feasible to develop protocols that are not only well-suited for real-world applications but also effectively address privacy requirements.
Get involved with the project!
Follow us on GitHub and Twitter
- GitHub: https://github.com/tiktok-privacy-innovation/PrivacyGo
- Twitter: https://twitter.com/TikTokForDevs
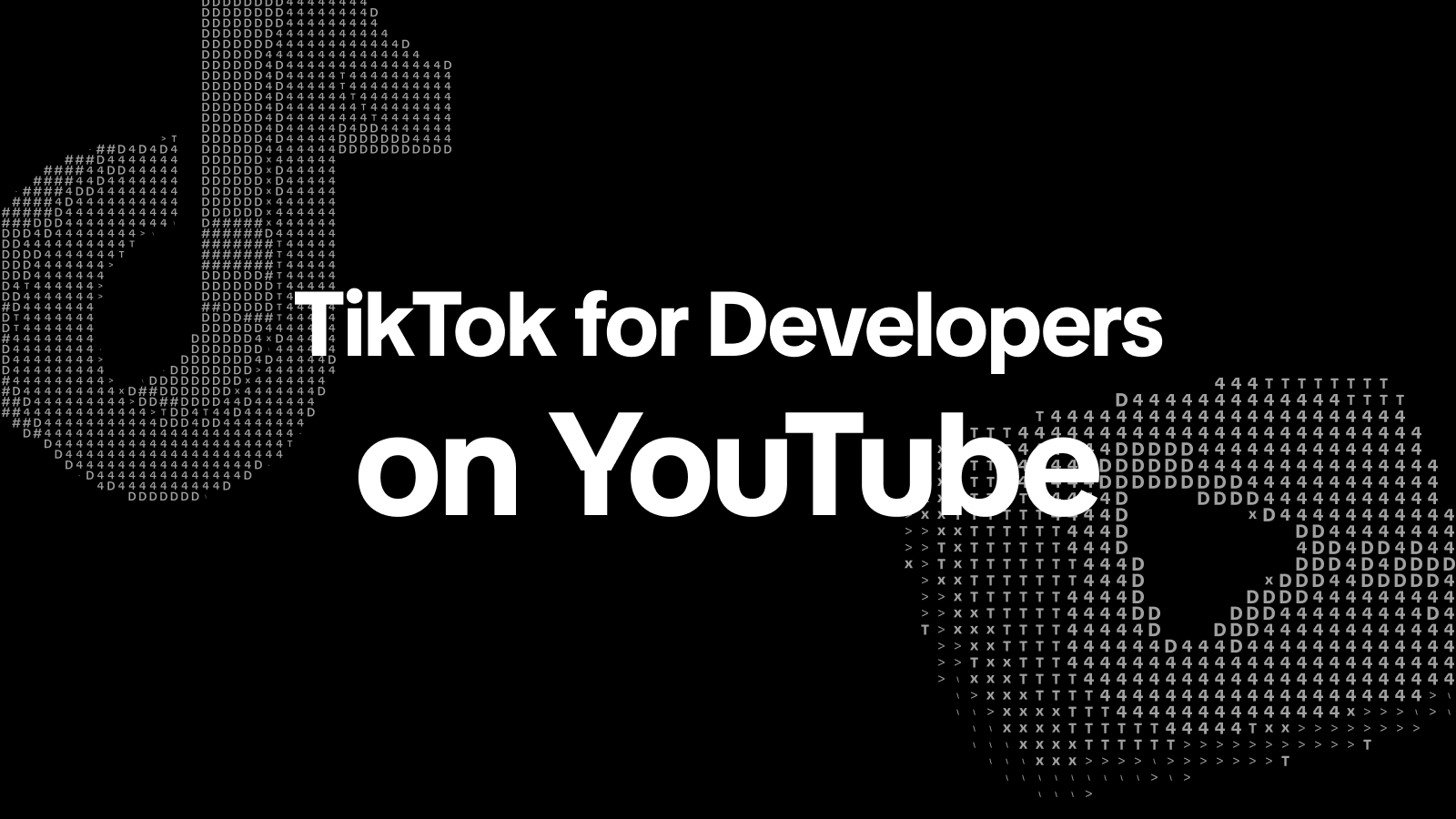
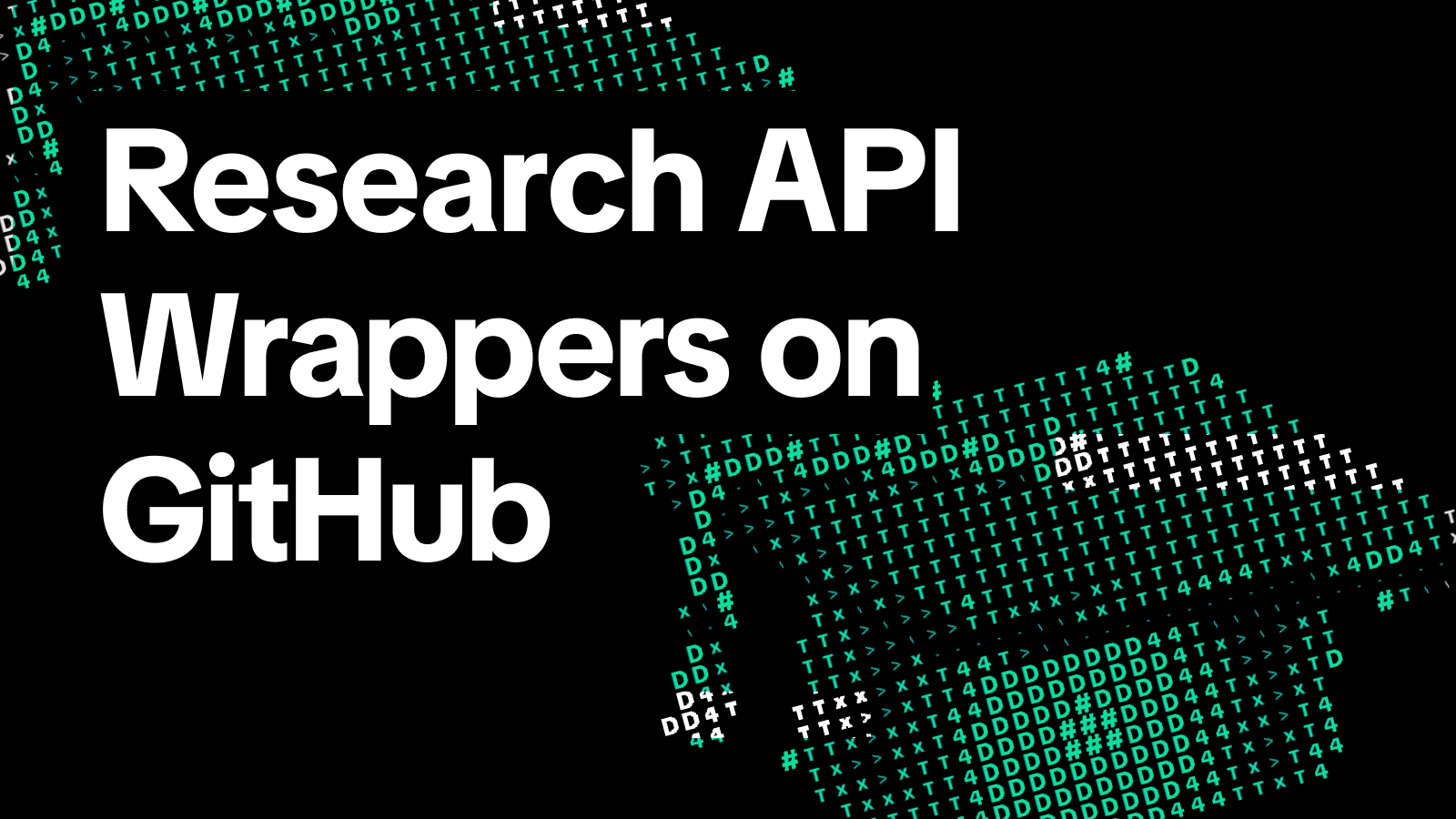
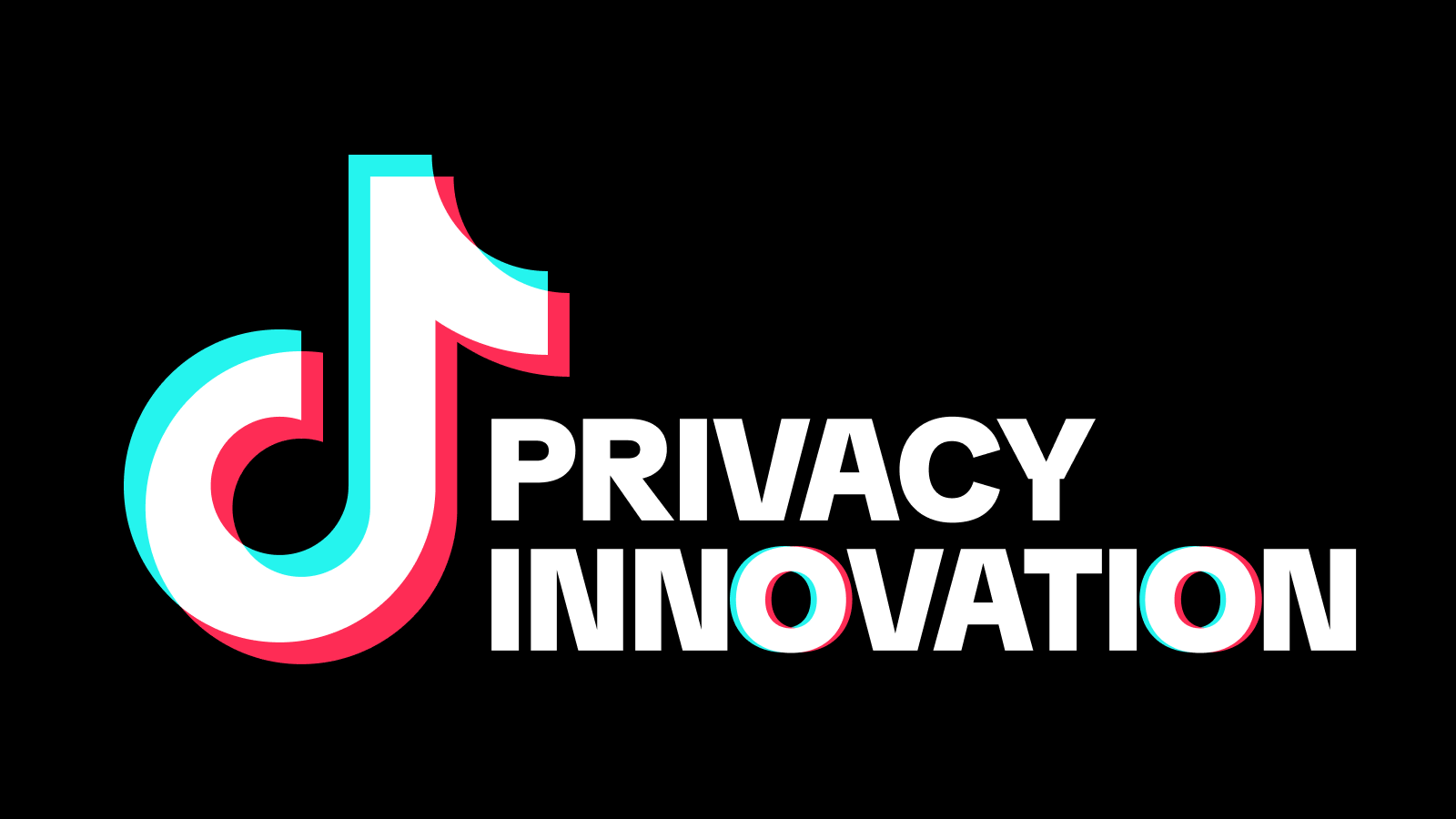